Divide-and-Conquer: Modality-aware Triple-Decoder Network for Robust RGB-T Salient Object Detection
2 Hong Kong University of Science and Technology, Hong Kong, China
3 Singapore Management University, Dalian, Singapore
Abstract
RGB-Thermal Salient Object Detection (RGB-T SOD) aims to pinpoint prominent objects within aligned pairs of visible and thermal infrared images. A key challenge lies in bridging the inherent disparities between RGB and Thermal modalities for effective saliency map prediction. Traditional encoder-decoder architectures, while designed for cross-modality feature interactions, may not have adequately considered the robustness against noise originating from defective modalities, thereby leading to suboptimal performance in complex scenarios. Inspired by hierarchical human visual systems, we propose the ConTriNet, a robust Confluent Triple-Flow Network employing a Divide-and-Conquer strategy. This framework utilizes a unified encoder with specialized decoders, each addressing different subtasks of exploring modality-specific and modality-complementary information for RGB-T SOD, thereby enhancing the final saliency map prediction. Specifically, ConTriNet comprises three flows: two modality-specific flows explore cues from RGB and Thermal modalities, and a third modality-complementary flow integrates cues from both modalities. ConTriNet presents several notable advantages. It incorporates a Modality-induced Feature Modulator (MFM) in the modality-shared union encoder to minimize inter-modality discrepancies and mitigate the impact of defective samples. Additionally, a foundational Residual Atrous Spatial Pyramid Module (RASPM) in the separated flows enlarges the receptive field, allowing for the capture of multi-scale contextual information. Furthermore, a Modality-aware Dynamic Aggregation Module (MDAM) in the modality-complementary flow dynamically aggregates saliency-related cues from both modality-specific flows. Leveraging the proposed parallel triple-flow framework, we further refine saliency maps derived from different flows through a flow-cooperative fusion strategy, yielding a high-quality, full-resolution saliency map for the final prediction. To evaluate the robustness and stability of our approach, we collect a comprehensive RGB-T SOD benchmark, VT-IMAG, covering various real-world challenging scenarios. Extensive experiments on public benchmarks and our VT-IMAG dataset confirm that ConTriNet consistently outperforms state-of-the-art competitors in both common and challenging scenarios, even when dealing with incomplete modality data.
Pipeline
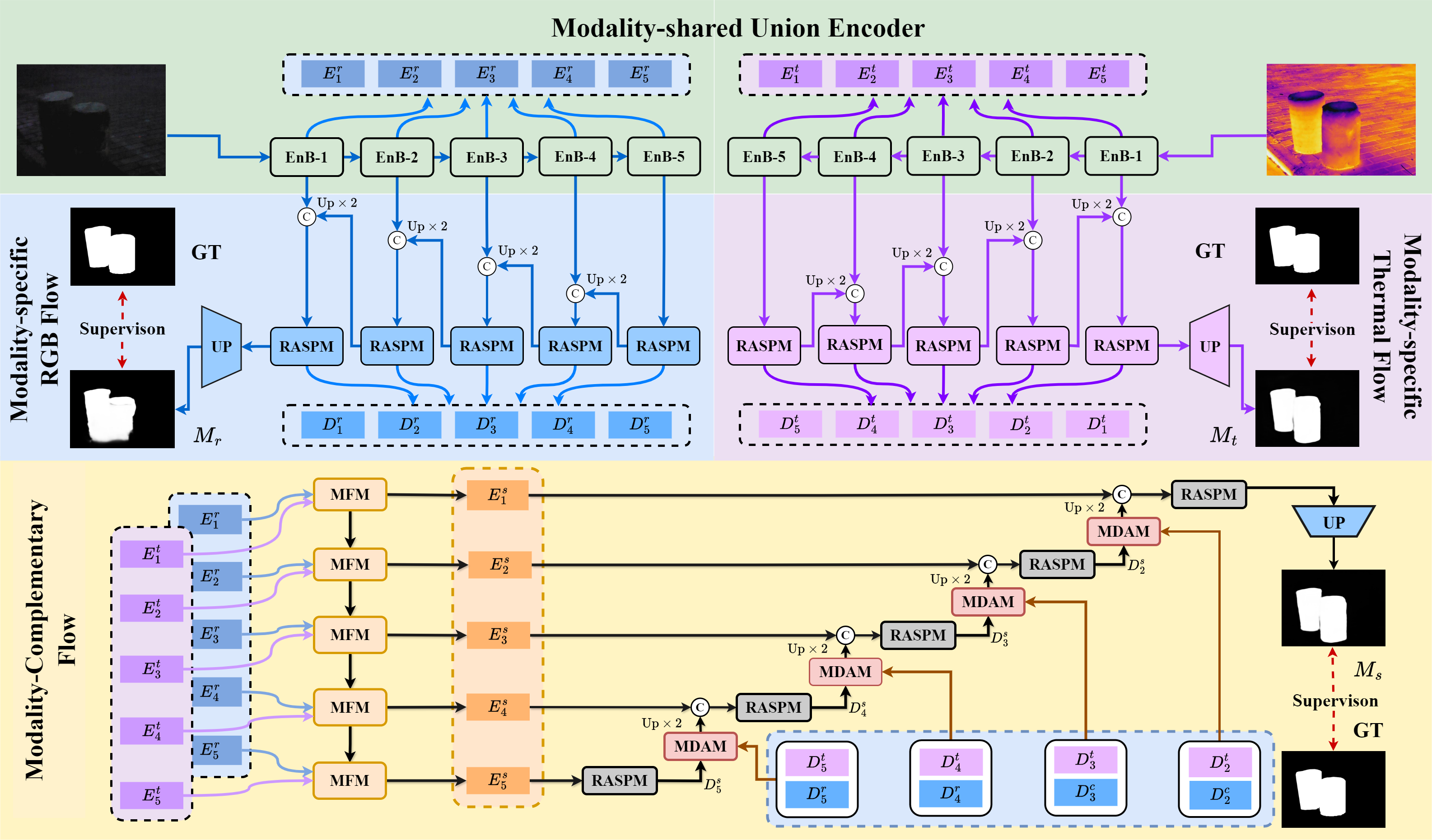
An overview of the proposed ConTriNet. ConTriNet comprises three main flows: a modality-complementary flow that predicts a modality-complementary saliency map, and two modality-specific flows that predict RGB- and Thermal-specific saliency maps, respectively.
Dataset Overview
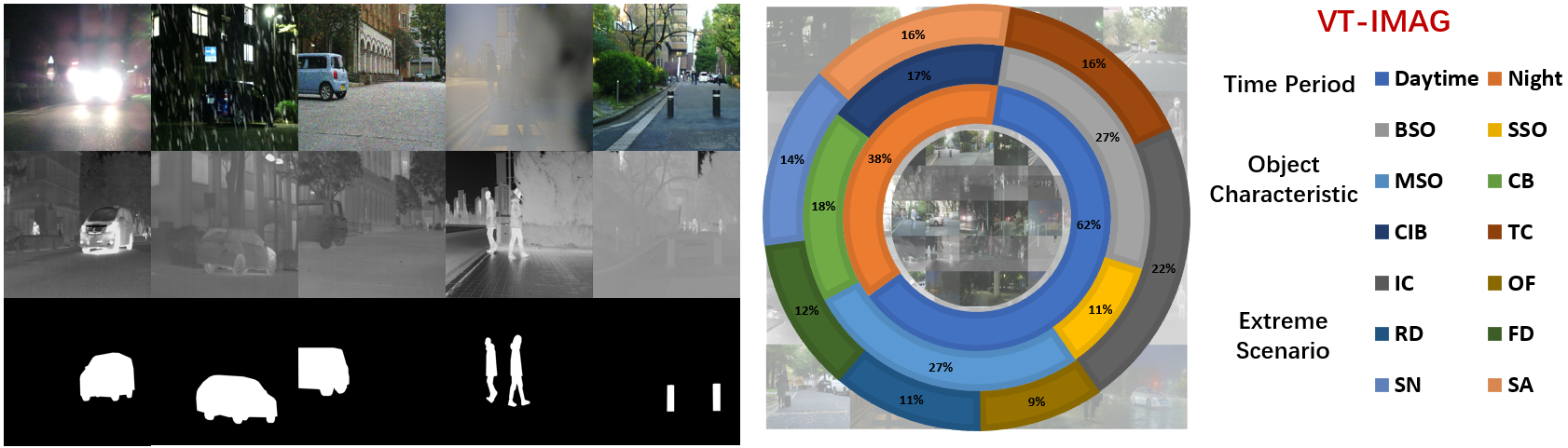
The primary purpose of the constructed VT-IMAG is to drive the advancement of RGB-T SOD methods and facilitate their deployment in real-world scenarios. For a fair comparison, all models are solely trained on clear data and simple scenes (i.e., training set of VT5000) and evaluated for Zero-shot Robustness on various real-world challenging cases in VT-IMAG.
Highlights
A novel Divide-and-Conquer strategy is introduced to address the weak robustness of current RGB-T SOD models. Within this context, we propose the ConTriNet, specified for effective fusing modality-complementary cues and deeply mining modality-specific cues.
An MFM is embedded in our union encoder to effectively narrow modality discrepancies and filter redundant or potentially disruptive information. Concurrently, an MDAM has been engineered into our modality-complementary flow to dynamically prioritize salient-related features from modality-specific flows while mitigating salient bias.
We present the RASPM, a cornerstone for our parallel flows that offers a larger yet compact receptive field. Stemming from this, we further introduce a flow-cooperative fusion strategy, aiming for a refined, comprehensive saliency map prediction.
A comprehensive benchmark with various challenging scenarios is contributed to the field as a new testbed for the faithful RGB-T SOD evaluations.
Quantitative Evaluation
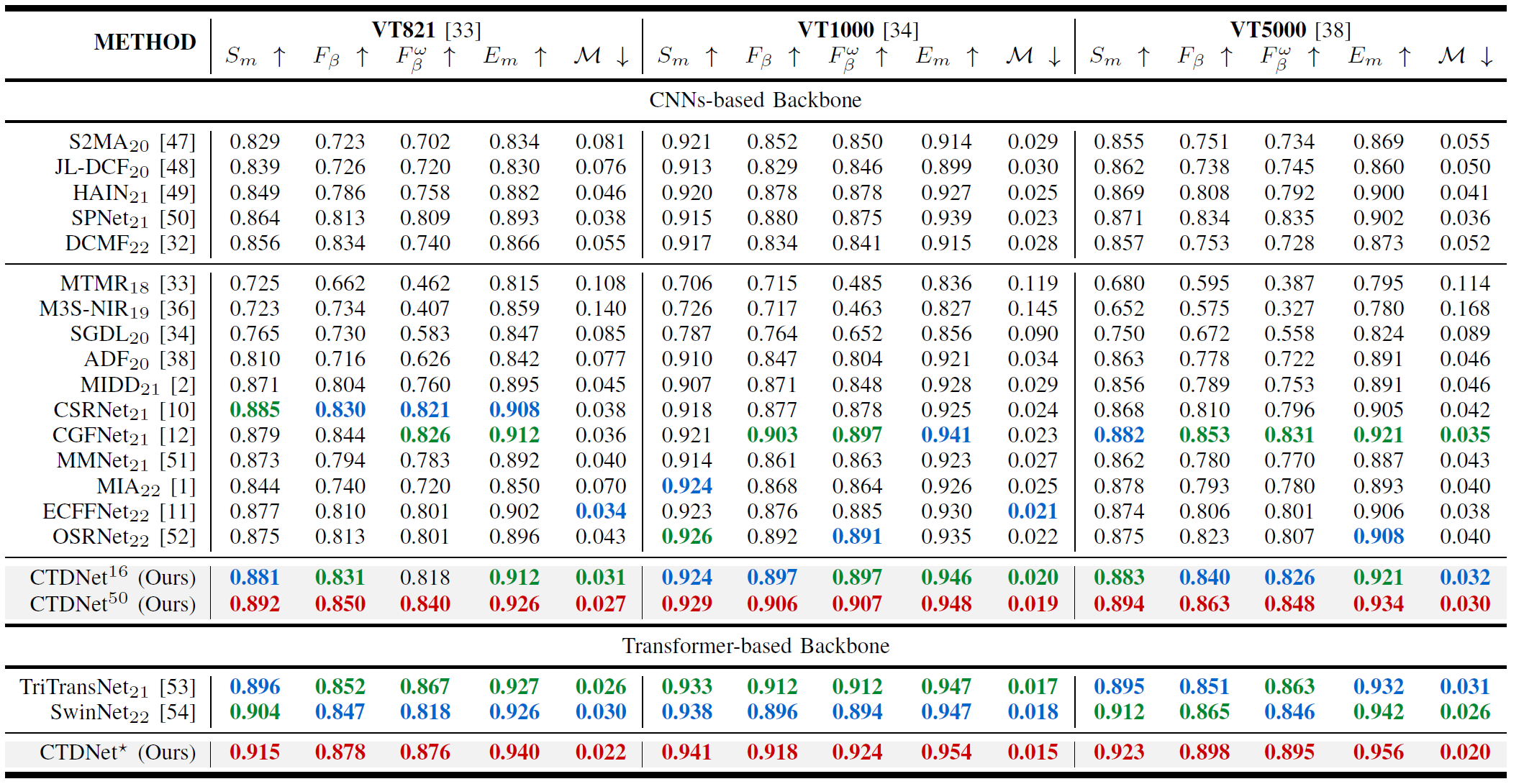
Comparison with recent state-of-the-art RGB-D/RGB-T SOD methods on VT821, VT1000 and VT5000. The top three results are highlighted using red, green and blue in the order. ConTriNet16 and ConTriNet50 indicate that using VGG backbone and ResNet backbone as the encoder, respectively. ConTriNet* indicate that using Swin-Transformer as the encoder.
Qualitative Evaluation
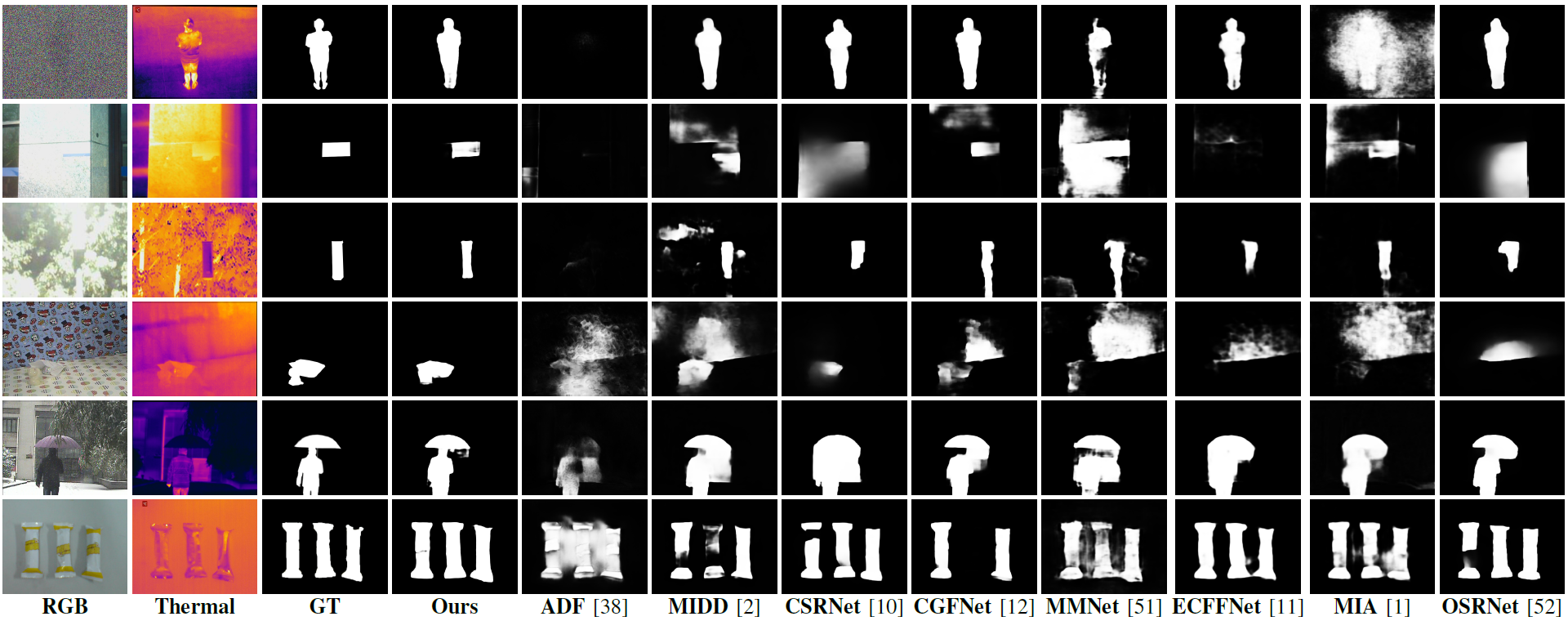
Visual examples of different methods on public datasets. From the left to right columns are RGB image, Thermal image, Ground-Truth and the results of eight state-of-the-art RGB-T SOD methods, respectively.
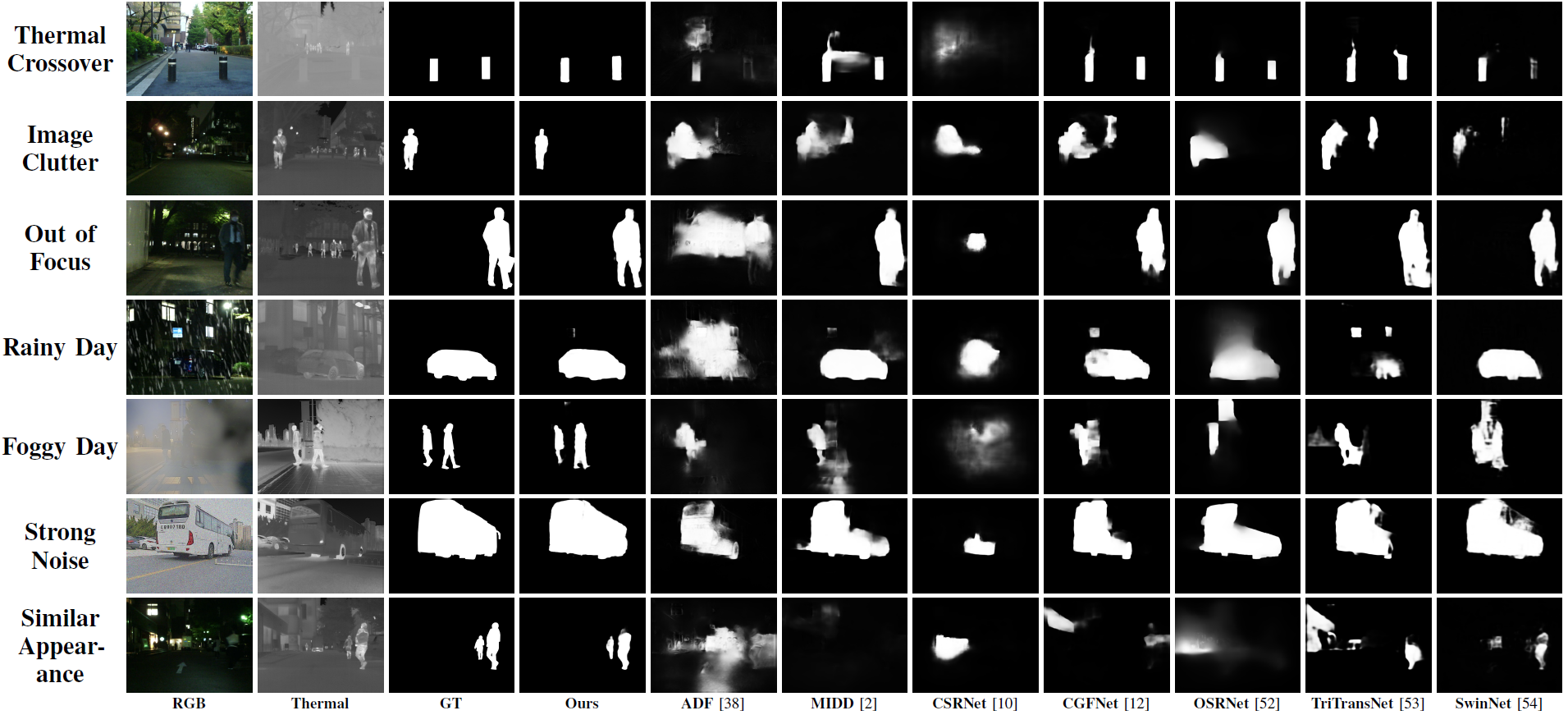
Qualitative comparisons of our proposed method and recent publicly available SOTA methods on various challenges in the proposed VT-IMAG.
Citation
@ARTICLE{10778650, author={Tang, Hao and Li, Zechao and Zhang, Dong and He, Shengfeng and Tang, Jinhui}, journal={IEEE Transactions on Pattern Analysis and Machine Intelligence}, title={Divide-and-Conquer: Confluent Triple-Flow Network for RGB-T Salient Object Detection}, year={2024}, volume={}, number={}, pages={1-17}, doi={10.1109/TPAMI.2024.3511621}}
Contact
If you have any questions, please contact Hao Tang at howard.haotang@gmail.com.